Science behind neuromind
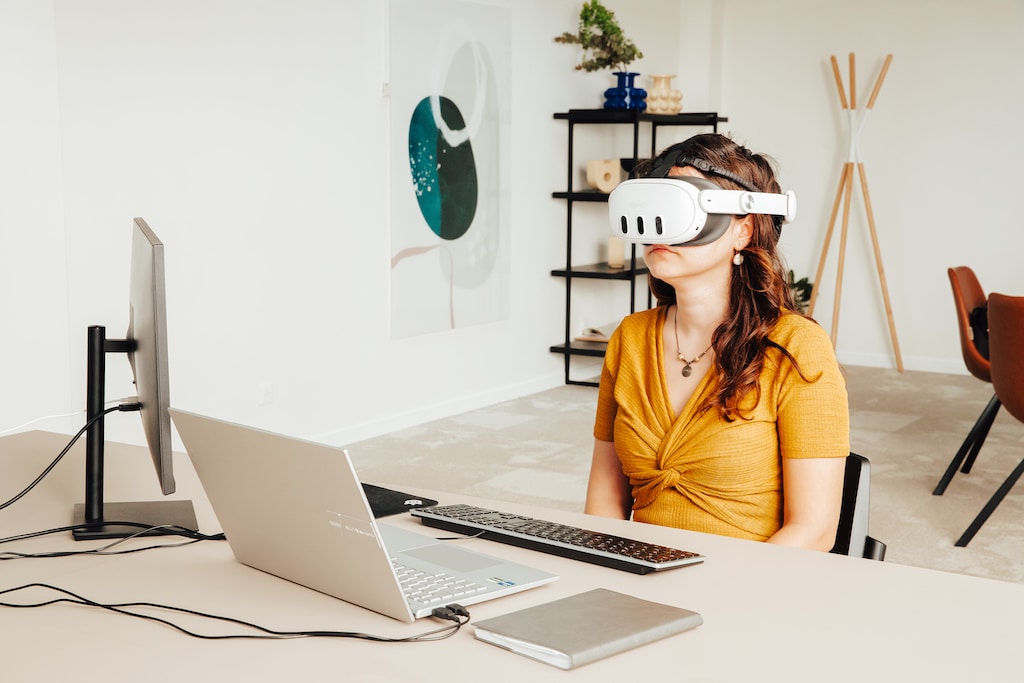
Neuromind solution
- 8 min read
Neuromind is based on several technologies and techniques that are already widely used and scientifically validated: neurofeedback, immersive virtual reality, and mindfulness-based therapies. By combining these three elements for the first time via proprietary biomarkers, we offer a novel solution for precision neurocognitive modulation.
Neurofeedback
Neurofeedback (NF) is a specific use case of brain-computer interfaces, aimed at teaching a user to voluntarily control their own brain activity. This learning process occurs via sensory feedback (usually visual-auditory) that reflects the real-time fluctuations of the target brain activity (direct or interpreted via biomarkers). When the user’s brain activity reaches or exceeds target values, positive feedback is generated. Otherwise, the feedback can be either negative or absent.
In neurofeedback protocols, a targeted neural activity that underlies a behavior, cognitive performance, or specific pathology is measured and compared in real-time to a predefined threshold (resting or baseline value). Neural activity can be measured via systems such as EEG, fMRI, or NIRS, and feedback indicating the measured intensity of the neural activity is provided to the participant in visual or auditory form. The participant self-regulates the targeted neural substrates based on the feedback.
A wide variety of neurofeedback training protocols have been applied to optimize performance. Observed benefits include sustained attention, orientation and executive attention, memory (including spatial memory), reaction time, complex psychomotor performance, implicit procedural memory, mood, and well-being.
Neurofeedback training is the subject of research within professional populations. The links between operational skills, cognitive abilities (attention skills, working memory), individuals’ perceptions (level of fatigue and mental workload), and the rhythms of brain electrical activity make neurofeedback training a cutting-edge tool conducive to the improvement and/or maintenance of cognitive abilities.
For example, a study demonstrated that it is possible to modify the average oscillation frequency of the Alpha range, progressively increasing it towards the upper limit of the frequency band (i.e., 12 Hz) in healthy subjects [1]. This particular neurofeedback training has shown very interesting potential for improving memory abilities (working memory) [2], [3], [4], as well as more specific skills such as precision task performance[5], which relies, among other things, on sustained attention (concentration). Other studies have used neurofeedback in Boundary Avoidance Tasks (BAT), showing that this tool could improve performance by nearly 25% on average by regulating vigilance levels in real-time [6]. Therefore, neurofeedback is a promising approach that can be used in a broader context of human performance optimization, particularly under conditions of intense and prolonged cognitive constraints [7].
Beyond performance optimization, neurofeedback has significant applications in clinical settings. It has been used effectively in the treatment of conditions like ADHD [8], [9], where individuals learn to regulate brain activity associated with attention and impulse control. NF has also shown promise in addressing anxiety, depression, and PTSD [10], helping patients modulate their emotional and cognitive responses. In these clinical applications, NF targets abnormal brainwave patterns, guiding the brain toward healthier states over time.
It has been demonstrated that the lasting effects of neurofeedback training are not only reflected in the ability to influence one’s own brain activity but are also related to behavioral changes or improvements in mental performance [11].
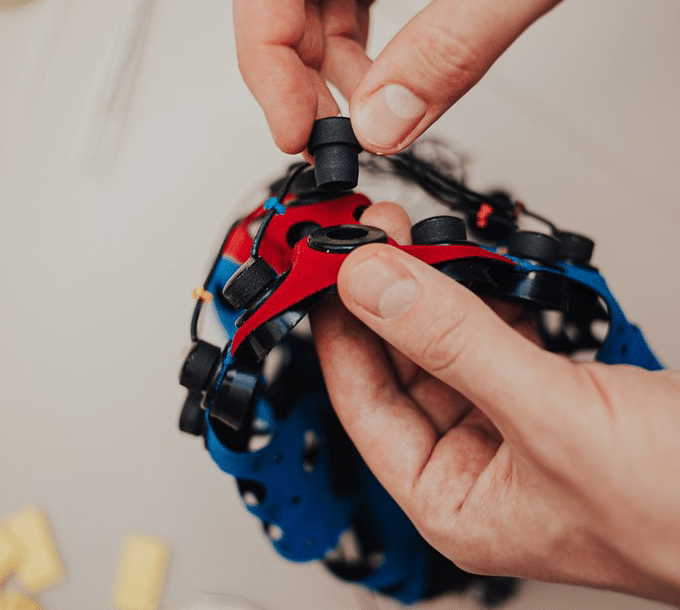
EEG electrodes used for neurofeedback
Mindfulness-based cognitive therapy (MBCT)
Mindfulness-Based Cognitive Therapy (MBCT) originated from Jon Kabat-Zinn’s stress reduction program at the University of Massachusetts Medical Center, initially developed to assist individuals with chronic pain and illness [12], [13].
In the 10 years since the publication of the MBCT manual, research has primarily been focused on addressing MBCT’s effectiveness. Data from six randomized controlled trials (n = 593) indicate that MBCT is associated with a 44% reduction in depressive relapse risk compared with usual care for patients with three or more previous episodes, and are as efficient as continuous antidepressant treatment (for those who keep adhered) [14]. More recent studies have shown that mindfulness based psychotherapies of 8 weeks prevent depressive relapses for 2 years [15]. Taking into account the high rate of adherence compared to antidepressant maintenance treatment with their adverse effects it makes psychotherapy the preferable option.
However, the applications of mindfulness have since expanded across a variety of fields. In education, mindfulness helps improve focus and emotional regulation in students; in corporate settings, it is used to reduce stress, enhance decision-making, and boost creativity; and in sports, it fosters mental clarity and resilience, optimizing performance.
While traditional MBCT involves an 8-week course of meditation practice, there is growing evidence that even single-session mindfulness interventions can yield tangible benefits [16]. Research has shown that brief mindfulness exercises can lead to immediate reductions in stress, improvements in mood, and enhanced cognitive function.
Nevertheless, conventional mindfulness-based interventions have their caveats too, such as the necessity of trained psychotherapists to guide it, overlap with the working hours or the absence of visual imagery or feedback to objectively estimate a patient’s mindfulness.
Here the recent technology advances, such as high-quality virtual reality and accessible easy-to-install physiological sensors come for help. The technology based implementation of mindfulness relies on the fact that mindfulness meditation is based on the 3 main components: attention control, emotion regulation and self-awareness, leading to eventual self-regulation [17]. The sustainable efficiency of mindfulness therapies implicates the mechanisms of neuroplasticity: the ability of the nervous system to durably modulate synaptic connections as a result of repetitive activation of the circuits [18].
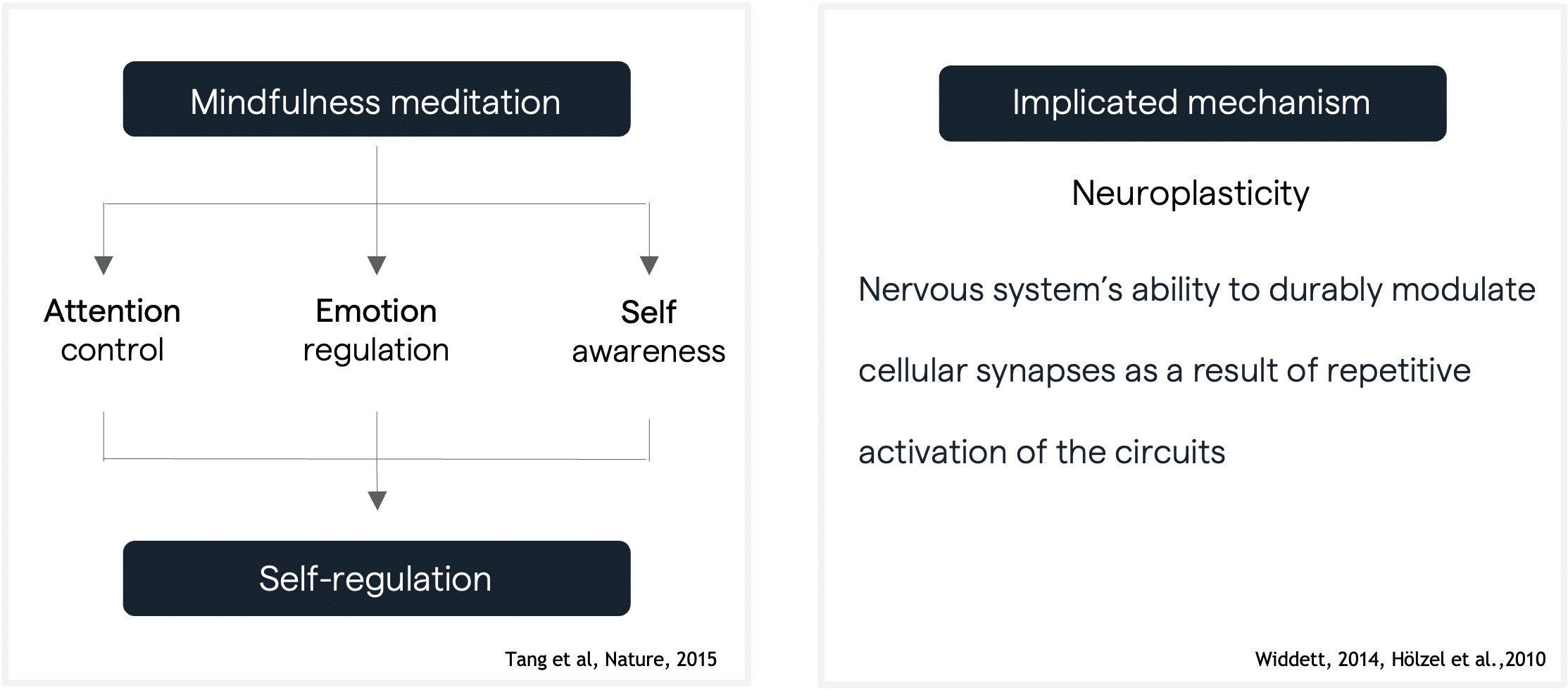
Mindfulness meditation working principles
Why combining Virtual Reality and Neurofeedback?
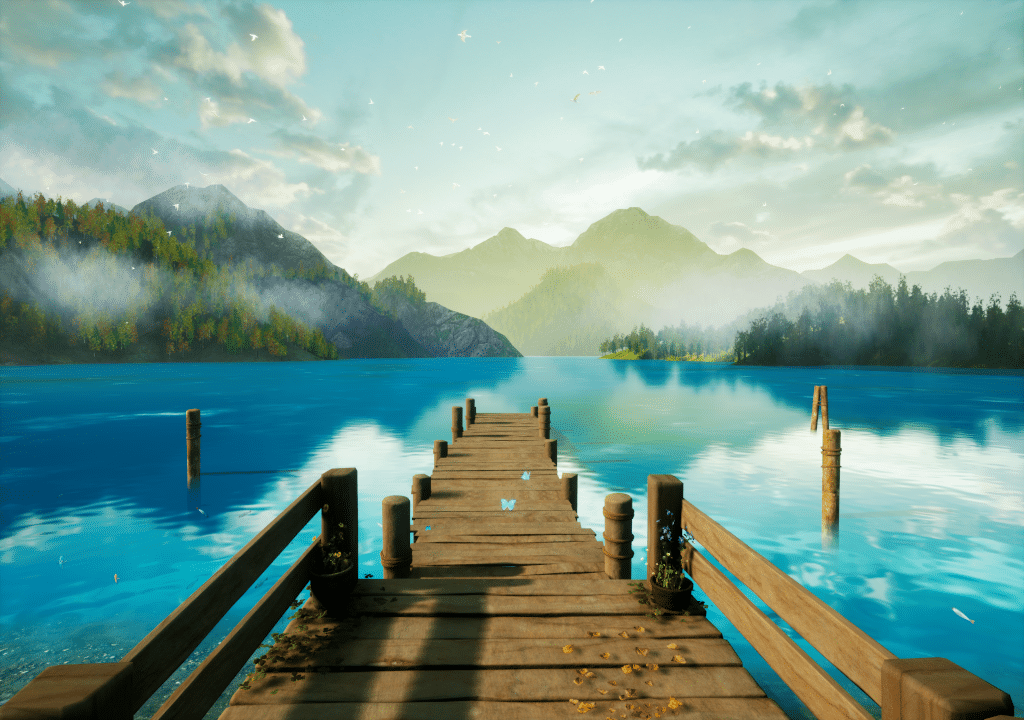
Lake meditation environment in Virtual Reality
These technologies then can play a crucial role in empowering mindfulness-based interventions in the following ways :
• VR provides sensory isolation and protects from external distractions, thus providing highly controlled environment; it increases adherence through gamification and it enables the first-time participants to reach the state of mindfulness faster due to visual and auditory immersion (no need to make an effort to imagine things) [19], [20]. The immersion of the patient in the virtual environment is accompanied by the feeling of “presence” which can be defined as the authentic feeling of existing in a world other than that where the body is physically located. This specificity, coupled with the ability to isolate the visual and auditory fields of the patient, has proven its effectiveness for the treatment of stress and anxiety [19], [22], [23].
• Neurofeedback provides real time emotion regulation and attention control, the pillars of meditation [21]; as well as real time personalized adaptation of the environment increasing engagement. There is growing evidence that interaction using neurofeedback can have benefits in terms of motivation, user experience, engagement, and attentional focus. [24].
Last but not the least, the neurofeedback provides a tool for therapy monitoring based on objective physiological data.
Although implementation of VR for mindfulness was already shown to be efficient [19], [20], [25], [26], [27] the introduction of biofeedback and neurofeedback is a very recent trend which may substantially improve the potential of autonomous mindfulness based interventions [21], [26], [28], [29], [30], [31], [32], [33], [34].
Thus it would fulfill the growing need for a digital psychotherapy tool with advantages of convenience, simplicity, low cost and high acceptability;
Affective Brain Computer Interface
Neuromind aims to develop an Affective Brain-Computer Interface (aBCI) that integrates emotion constructionist theory to implement a mindfulness-based approach into neurofeedback-driven virtual reality (VR). Brain-computer interfaces (BCIs) are typically defined as direct communication pathways between the brain and external devices, allowing brain signals to control external activities. In the context of aBCIs, these systems estimate human emotions or affect from brain signals, offering insight into the user’s emotional states.
Interest in the automatic detection of affective states has significantly grown in recent years, with research underscoring the vital role affect plays in human decision-making processes. Moreover, emotional regulation, which is closely linked to achieving mindfulness, can be enhanced through targeted neurofeedback approaches. The robust body of scientific research demonstrates the feasibility of detecting emotional signatures in EEG signals [35], [36], [37], where emotions are often modeled in terms of valence (ranging from negative to positive) and arousal (degree of intensity). This emotional mapping can be effectively captured with a modest number of EEG electrodes.
Additionally, the integration of eye-tracking technology in modern VR headsets allows for the monitoring of attentional control and arousal via pupillometry [38], [39], [40], further enhancing the precision of emotional detection in immersive environments.
These advancements offer promising applications for precision neurocognitive modulation through real-time, personalized emotional and attentional control…
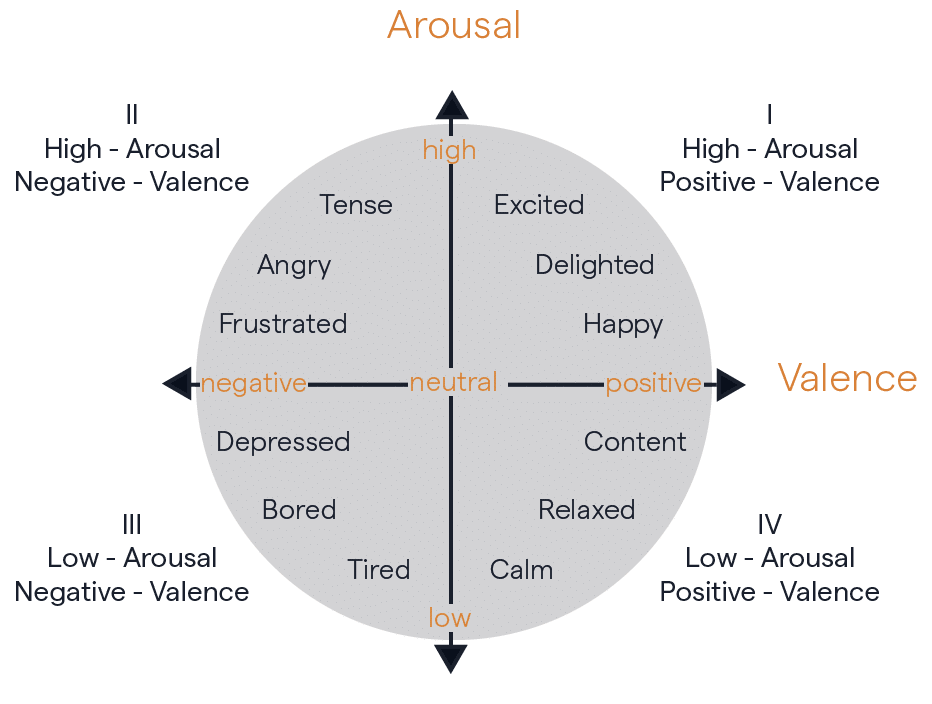
Emotion mapping of circumplex theory
Anton Filipchuk
Lead of scientific research, Healthy Mind
[1] B. Zoefel, R. J. Huster, and C. S. Herrmann, ‘Neurofeedback training of the upper alpha frequency band in EEG improves cognitive performance’, NeuroImage, vol. 54, no. 2, pp. 1427–1431, Jan. 2011, doi: 10.1016/j.neuroimage.2010.08.078.
[2] M. Brickwedde, M. C. Krüger, and H. R. Dinse, ‘Somatosensory alpha oscillations gate perceptual learning efficiency’, Nat. Commun., vol. 10, no. 1, p. 263, Jan. 2019, doi: 10.1038/s41467-018-08012-0.
[3] C. Escolano, M. Aguilar, and J. Minguez, ‘EEG-based upper alpha neurofeedback training improves working memory performance’, in 2011 Annual International Conference of the IEEE Engineering in Medicine and Biology Society, Boston, MA: IEEE, Aug. 2011, pp. 2327–2330. doi: 10.1109/IEMBS.2011.6090651.
[4] Y. Lavy, T. Dwolatzky, Z. Kaplan, J. Guez, and D. Todder, ‘Neurofeedback Improves Memory and Peak Alpha Frequency in Individuals with Mild Cognitive Impairment’, Appl. Psychophysiol. Biofeedback, vol. 44, no. 1, pp. 41–49, Mar. 2019, doi: 10.1007/s10484-018-9418-0.
[5] Y. Liu, S. C. Harihara Subramaniam, O. Sourina, E. Shah, J. Chua, and K. Ivanov, ‘NeuroFeedback Training for Enhancement of the Focused Attention Related to Athletic Performance in Elite Rifle Shooters’, in Transactions on Computational Science XXXII, vol. 10830, M. L. Gavrilova, C. J. K. Tan, and A. Sourin, Eds., in Lecture Notes in Computer Science, vol. 10830. , Berlin, Heidelberg: Springer Berlin Heidelberg, 2018, pp. 106–119. doi: 10.1007/978-3-662-56672-5_8.
[6] J. Faller, J. Cummings, S. Saproo, and P. Sajda, ‘Regulation of arousal via online neurofeedback improves human performance in a demanding sensory-motor task’, Proc. Natl. Acad. Sci., vol. 116, no. 13, pp. 6482–6490, Mar. 2019, doi: 10.1073/pnas.1817207116.
[7] F. Dehais, A. Lafont, R. Roy, and S. Fairclough, ‘A Neuroergonomics Approach to Mental Workload, Engagement and Human Performance’, Front. Neurosci., vol. 14, p. 268, Apr. 2020, doi: 10.3389/fnins.2020.00268.
[8] M. Arns and J. L. Kenemans, ‘Neurofeedback in ADHD and insomnia: Vigilance stabilization through sleep spindles and circadian networks’, Neurosci. Biobehav. Rev., vol. 44, pp. 183–194, Jul. 2014, doi: 10.1016/j.neubiorev.2012.10.006.
[9] L. E. Arnold et al., ‘EEG Neurofeedback for ADHD: Double-Blind Sham-Controlled Randomized Pilot Feasibility Trial’, J. Atten. Disord., vol. 17, no. 5, pp. 410–419, Jul. 2013, doi: 10.1177/1087054712446173.
[10] B. A. Van Der Kolk et al., ‘A Randomized Controlled Study of Neurofeedback for Chronic PTSD’, PLOS ONE, vol. 11, no. 12, p. e0166752, Dec. 2016, doi: 10.1371/journal.pone.0166752.
[11] A. Caria, R. Sitaram, and N. Birbaumer, ‘Real-Time fMRI: A Tool for Local Brain Regulation’, The Neuroscientist, vol. 18, no. 5, pp. 487–501, Oct. 2012, doi: 10.1177/1073858411407205.
[12] J. M. G. Williams and W. Kuyken, ‘Mindfulness-based cognitive therapy: a promising new approach to preventing depressive relapse’, Br. J. Psychiatry, vol. 200, no. 5, pp. 359–360, May 2012, doi: 10.1192/bjp.bp.111.104745.
[13] J. Kabat-Zinn, Full catastrophe living: how to cope with stress, pain and illness using mindfulness meditation, Revised and Updated edition. London: Piatkus, 2020.
[14] J. Piet and E. Hougaard, ‘The effect of mindfulness-based cognitive therapy for prevention of relapse in recurrent major depressive disorder: A systematic review and meta-analysis’, Clin. Psychol. Rev., vol. 31, no. 6, pp. 1032–1040, Aug. 2011, doi: 10.1016/j.cpr.2011.05.002.
[15] W. Kuyken et al., ‘Effectiveness and cost-effectiveness of mindfulness-based cognitive therapy compared with maintenance antidepressant treatment in the prevention of depressive relapse or recurrence (PREVENT): a randomised controlled trial’, The Lancet, vol. 386, no. 9988, pp. 63–73, Jul. 2015, doi: 10.1016/S0140-6736(14)62222-4.
[16] A. Berkovich-Ohana, J. Glicksohn, and A. Goldstein, ‘Mindfulness-induced changes in gamma band activity – Implications for the default mode network, self-reference and attention’, Clin. Neurophysiol., vol. 123, no. 4, pp. 700–710, Apr. 2012, doi: 10.1016/j.clinph.2011.07.048.
[17] Y.-Y. Tang, B. K. Hölzel, and M. I. Posner, ‘The neuroscience of mindfulness meditation’, Nat. Rev. Neurosci., vol. 16, no. 4, pp. 213–225, Apr. 2015, doi: 10.1038/nrn3916.
[18] B. K. Hölzel et al., ‘Mindfulness practice leads to increases in regional brain gray matter density’, Psychiatry Res. Neuroimaging, vol. 191, no. 1, pp. 36–43, Jan. 2011, doi: 10.1016/j.pscychresns.2010.08.006.
[19] M. V. Navarro-Haro et al., ‘Evaluation of a Mindfulness-Based Intervention With and Without Virtual Reality Dialectical Behavior Therapy® Mindfulness Skills Training for the Treatment of Generalized Anxiety Disorder in Primary Care: A Pilot Study’, Front. Psychol., vol. 10, p. 55, Jan. 2019, doi: 10.3389/fpsyg.2019.00055.
[20] M. V. Navarro-Haro et al., ‘Meditation experts try Virtual Reality Mindfulness: A pilot study evaluation of the feasibility and acceptability of Virtual Reality to facilitate mindfulness practice in people attending a Mindfulness conference.’, PLOS ONE, vol. 12, no. 11, p. e0187777, Nov. 2017, doi: 10.1371/journal.pone.0187777.
[21] D. A. Ziegler et al., ‘Closed-loop digital meditation improves sustained attention in young adults’, Nat. Hum. Behav., vol. 3, no. 7, pp. 746–757, Jul. 2019, doi: 10.1038/s41562-019-0611-9.
[22] A. P. Anderson, M. D. Mayer, A. M. Fellows, D. R. Cowan, M. T. Hegel, and J. C. Buckey, ‘Relaxation with Immersive Natural Scenes Presented Using Virtual Reality’, Aerosp. Med. Hum. Perform., vol. 88, no. 6, pp. 520–526, Jun. 2017, doi: 10.3357/AMHP.4747.2017.
[23] D. Villani, F. Riva, and G. Riva, ‘New technologies for relaxation: The role of presence.’, Int. J. Stress Manag., vol. 14, no. 3, pp. 260–274, Aug. 2007, doi: 10.1037/1072-5245.14.3.260.
[24] R. Lüddecke and A. Felnhofer, ‘Virtual Reality Biofeedback in Health: A Scoping Review’, Appl. Psychophysiol. Biofeedback, vol. 47, no. 1, pp. 1–15, Mar. 2022, doi: 10.1007/s10484-021-09529-9.
[25] N. Döllinger, C. Wienrich, and M. E. Latoschik, ‘Challenges and Opportunities of Immersive Technologies for Mindfulness Meditation: A Systematic Review’, Front. Virtual Real., vol. 2, p. 644683, Apr. 2021, doi: 10.3389/frvir.2021.644683.
[26] I. Kosunen, M. Salminen, S. Järvelä, A. Ruonala, N. Ravaja, and G. Jacucci, ‘RelaWorld: Neuroadaptive and Immersive Virtual Reality Meditation System’, in Proceedings of the 21st International Conference on Intelligent User Interfaces, Sonoma California USA: ACM, Mar. 2016, pp. 208–217. doi: 10.1145/2856767.2856796.
[27] E. Seabrook et al., ‘Understanding How Virtual Reality Can Support Mindfulness Practice: Mixed Methods Study’, J. Med. Internet Res., vol. 22, no. 3, p. e16106, Mar. 2020, doi: 10.2196/16106.
[28] A. Choo and A. May, ‘Virtual mindfulness meditation: Virtual reality and electroencephalography for health gamification’, in 2014 IEEE Games Media Entertainment, Toronto, ON: IEEE, Oct. 2014, pp. 1–3. doi: 10.1109/GEM.2014.7048076.
[29] M. Cuesta et al., ‘Virtual Reality and EEG-Based Intelligent Agent in Older Adults With Subjective Cognitive Decline: A Feasibility Study for Effects on Emotion and Cognition’, Front. Virtual Real., vol. 2, p. 807991, Jan. 2022, doi: 10.3389/frvir.2021.807991.
[30] X. Tong, D. Gromala, A. Choo, A. Amin, and C. Shaw, ‘The Virtual Meditative Walk: An Immersive Virtual Environment for Pain Self-modulation Through Mindfulness-Based Stress Reduction Meditation’, in Virtual, Augmented and Mixed Reality, vol. 9179, R. Shumaker and S. Lackey, Eds., in Lecture Notes in Computer Science, vol. 9179. , Cham: Springer International Publishing, 2015, pp. 388–397. doi: 10.1007/978-3-319-21067-4_40.
[31] P. A. Bloom et al., ‘Mindfulness-based real-time fMRI neurofeedback: a randomized controlled trial to optimize dosing for depressed adolescents’, BMC Psychiatry, vol. 23, no. 1, p. 757, Oct. 2023, doi: 10.1186/s12888-023-05223-8.
[32] J. Zhang et al., ‘Reducing default mode network connectivity with mindfulness-based fMRI neurofeedback: a pilot study among adolescents with affective disorder history’, Mol. Psychiatry, vol. 28, no. 6, pp. 2540–2548, Jun. 2023, doi: 10.1038/s41380-023-02032-z.
[33] T. Chow, T. Javan, T. Ros, and P. Frewen, ‘EEG Dynamics of Mindfulness Meditation Versus Alpha Neurofeedback: a Sham-Controlled Study’, Mindfulness, vol. 8, no. 3, pp. 572–584, Jun. 2017, doi: 10.1007/s12671-016-0631-8.
[34] H. Hunkin, D. L. King, and I. T. Zajac, ‘EEG Neurofeedback During Focused Attention Meditation: Effects on State Mindfulness and Meditation Experiences’, Mindfulness, vol. 12, no. 4, pp. 841–851, Apr. 2021, doi: 10.1007/s12671-020-01541-0.
[35] M. Wyczesany and T. S. Ligeza, ‘Towards a constructionist approach to emotions: verification of the three-dimensional model of affect with EEG-independent component analysis’, Exp. Brain Res., vol. 233, no. 3, pp. 723–733, Mar. 2015, doi: 10.1007/s00221-014-4149-9.
[36] L. Shu et al., ‘A Review of Emotion Recognition Using Physiological Signals’, Sensors, vol. 18, no. 7, p. 2074, Jun. 2018, doi: 10.3390/s18072074.
[37] J. Marín-Morales et al., ‘Affective computing in virtual reality: emotion recognition from brain and heartbeat dynamics using wearable sensors’, Sci. Rep., vol. 8, no. 1, p. 13657, Dec. 2018, doi: 10.1038/s41598-018-32063-4.
[38] A. Widmann, E. Schröger, and N. Wetzel, ‘Emotion lies in the eye of the listener: Emotional arousal to novel sounds is reflected in the sympathetic contribution to the pupil dilation response and the P3’, Biol. Psychol., vol. 133, pp. 10–17, Mar. 2018, doi: 10.1016/j.biopsycho.2018.01.010.
[39] M. M. Bradley, L. Miccoli, M. A. Escrig, and P. J. Lang, ‘The pupil as a measure of emotional arousal and autonomic activation’, Psychophysiology, vol. 45, no. 4, pp. 602–607, Jul. 2008, doi: 10.1111/j.1469-8986.2008.00654.x.
[40] J. Z. Lim, J. Mountstephens, and J. Teo, ‘Emotion Recognition Using Eye-Tracking: Taxonomy, Review and Current Challenges’, Sensors, vol. 20, no. 8, p. 2384, Apr. 2020, doi: 10.3390/s20082384.